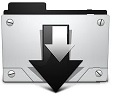
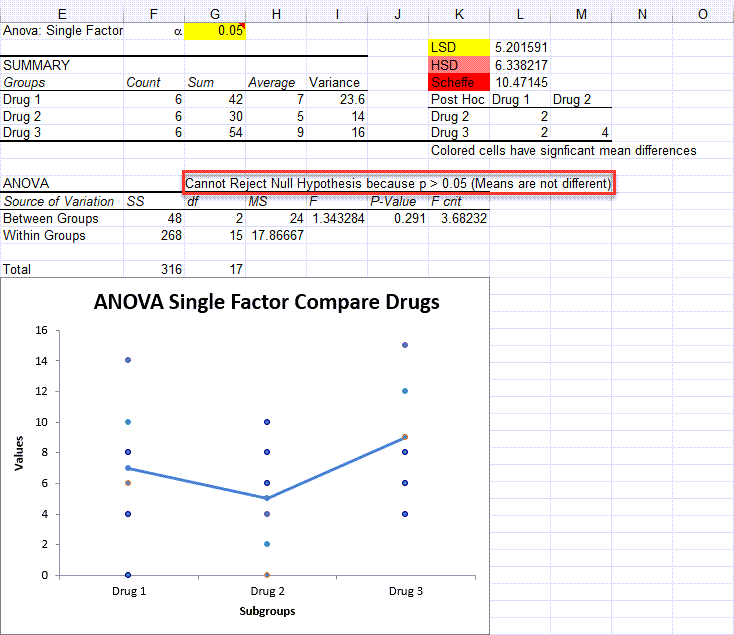
The top part of Figure 3 contains the rest of the output from the data analysis tool.
#Two way anova in excel with replication how to
We now return to Example 1 and show how to conduct the required analysis using Excel’s Anova: Two-factor With Replication data analysis tool.Įxample 1 (continued): The summary output from the data analysis tool is given on the right side of Figure 2, with the sample data repeated on the left side of the figure.įigure 2 – Summary output of ANOVA data analysis for Example 1

If all μ i are equal and all are equal then Theorem 1: Suppose a sample is made as described in Definitions 1 and 2, with the x ijk independently and normally distributed. Proof: The proof is similar to that of Property 1 of Basic Concepts for ANOVA. Property 3: If a sample is made as described in Definition 1 and 2, with the x ijkindependently and normally distributed and with all (or ) equal, then The proof is similar to the proof of Property 1. Property 2: Note that the between-group terms are as for the one-way ANOVA, namely If we square both sides of the equation, sum over i, j and k, and then simplify (with various terms equal to zero as in the proof of Property 2 of Basic Concepts for ANOVA), we get the first result. Since the within groups terms are used as the error terms in our model, we also use the following symbols: We can also define the following entities: In addition, there is a null hypothesis for the effects due to the interaction between factors A and B.ĭefinition 2: Using the terminology of Definition 1, define Where e ijkis the counterpart to ε ijkin the sample. Note thatĪs in Definition 1 of Two Factor ANOVA without Replication, the null hypotheses for the main effects are: Where ε ijk denotes the error (or unexplained) amount. Similarly, we haveįinally, we can represent each element in the sample as the interaction of level i of factor A and level j of factor B. We use δ ijfor the effect of level i of factor A with level j of factor B, i.e. Īs in Definition 1 of Two Factor ANOVA without Replication, we define the effects α i and β j where In Definition 1 of Two Factor ANOVA without Replication the r × c table contains the entries.

As usual, we start with an example.Įxample 1: Repeat the analysis from Example 1 of Two Factor ANOVA without Replication, but this time with the data shown in Figure 1 where each combination of blend and crop has a sample of size 5.ĭefinition 1: We extend the structural model of Definition 1 of Two Factor ANOVA without Replication as follows. In Unbalanced Factorial ANOVA we show how to perform the analysis where the samples are not equal ( unbalanced model) via regression. We will restrict ourselves to the case where all the samples are equal in size ( balanced model). Note that ANOVA with replication should not be confused with ANOVA with repeated measures as described at ANOVA with Repeated Measures. We now consider Two-factor ANOVA with replication where there is more than one sample element for each combination of factor A levels and factor B levels. In Two Factor ANOVA without Replication there was only one sample item for each combination of factor A levels and factor B levels.
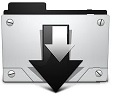